Comparing layers: a practical case on Fashion Mnist¶
In this tutorial, we compare the performance of different layers for fashion mnist dataset
import tensorflow
import numpy as np
import matplotlib.pyplot as plt
plt.rc('font', family='serif')
plt.rc('xtick', labelsize='x-small')
plt.rc('ytick', labelsize='x-small')
# Model / data parameters
num_classes = 10
input_shape = (28, 28, 1)
#use_samples=256
use_samples=1024
# the data, split between train and test sets
(x_train, y_train), (x_test, y_test) = tensorflow.keras.datasets.fashion_mnist.load_data()
x_train=x_train[0:use_samples]
y_train=y_train[0:use_samples]
# Scale images to the [0, 1] range
x_train = x_train.astype("float32") / 255
x_test = x_test.astype("float32") / 255
# Make sure images have shape (28, 28, 1)
x_train = np.expand_dims(x_train, -1)
x_test = np.expand_dims(x_test, -1)
print("x_train shape:", x_train.shape)
print(x_train.shape[0], "train samples")
print(x_test.shape[0], "test samples")
# convert class vectors to binary class matrices
y_train = tensorflow.keras.utils.to_categorical(y_train, num_classes)
y_test = tensorflow.keras.utils.to_categorical(y_test, num_classes)
x_train shape: (1024, 28, 28, 1)
1024 train samples
10000 test samples
from morpholayers import *
from morpholayers.layers import *
from morpholayers.constraints import *
from morpholayers.regularizers import *
from tensorflow.keras.layers import Input,Conv2D,MaxPooling2D,Flatten,Dropout,Dense
from tensorflow.keras.models import Model
batch_size = 128
epochs = 40
nfilterstolearn=8
filter_size=5
regularizer_parameter=.002
from sklearn.metrics import classification_report,confusion_matrix
def get_model(layer0):
xin=Input(shape=input_shape)
xlayer=layer0(xin)
x=MaxPooling2D(pool_size=(2, 2))(xlayer)
x=Conv2D(32, kernel_size=(3, 3), activation="relu")(x)
x=MaxPooling2D(pool_size=(2, 2))(x)
x=Flatten()(x)
x=Dropout(0.5)(x)
xoutput=Dense(num_classes, activation="softmax")(x)
return Model(xin,outputs=xoutput), Model(xin,outputs=xlayer)
def plot_history(history):
plt.figure()
plt.plot(history.history['loss'],label='loss')
plt.plot(history.history['val_loss'],label='val_loss')
plt.xlabel('Epochs')
plt.ylabel('Loss')
plt.grid('on')
plt.legend()
plt.show()
plt.plot(history.history['accuracy'],label='acc')
plt.plot(history.history['val_accuracy'],label='val_acc')
plt.grid('on')
plt.xlabel('Epochs')
plt.ylabel('Accuracy')
plt.legend()
plt.show()
def plot_output_filters(model):
fig=plt.figure()
Z=model.predict(x_train[0:1,:,:,:])
for i in range(Z.shape[3]):
plt.subplot(2,Z.shape[3]/2,i+1)
plt.imshow(Z[0,:,:,i],cmap='gray',vmax=Z.max(),vmin=Z.min())
#plt.colorbar()
fig.suptitle('Output of Learned Filters for an example')
def plot_filters(model):
Z=model.layers[-1].get_weights()[0]
fig=plt.figure()
for i in range(Z.shape[3]):
plt.subplot(2,Z.shape[3]/2,i+1)
plt.imshow(Z[:,:,0,i],cmap='RdBu',vmax=Z.max(),vmin=Z.min())
fig.suptitle('Learned Filters')
def see_results_layer(layer,lr=.001):
modeli,modellayer=get_model(layer)
modeli.summary()
modeli.compile(loss="categorical_crossentropy", optimizer=tensorflow.keras.optimizers.Adam(lr=lr), metrics=["accuracy"])
historyi=modeli.fit(x_train, y_train, batch_size=batch_size, epochs=epochs, validation_data=(x_test,y_test),
callbacks=[tf.keras.callbacks.EarlyStopping(monitor='loss', patience=5,restore_best_weights=True),
tf.keras.callbacks.ReduceLROnPlateau(patience=2,factor=.5)],verbose=0)
Y_test = np.argmax(y_test, axis=1) # Convert one-hot to index
y_pred = np.argmax(modeli.predict(x_test),axis=1)
CM=confusion_matrix(Y_test, y_pred)
print(CM)
plt.imshow(CM,cmap='hot',vmin=0,vmax=1000)
plt.title('Confusion Matrix')
plt.show()
print(classification_report(Y_test, y_pred))
plot_history(historyi)
plot_filters(modellayer)
plot_output_filters(modellayer)
return historyi
Example of Classical Convolutional Layer¶
histConv=see_results_layer(Conv2D(nfilterstolearn, kernel_size=(filter_size, filter_size),
kernel_regularizer=tensorflow.keras.regularizers.l1_l2(l1=regularizer_parameter,l2=regularizer_parameter), activation="relu"),lr=.01)
Model: "model"
_________________________________________________________________
Layer (type) Output Shape Param #
=================================================================
input_1 (InputLayer) [(None, 28, 28, 1)] 0
_________________________________________________________________
conv2d (Conv2D) (None, 24, 24, 8) 208
_________________________________________________________________
max_pooling2d (MaxPooling2D) (None, 12, 12, 8) 0
_________________________________________________________________
conv2d_1 (Conv2D) (None, 10, 10, 32) 2336
_________________________________________________________________
max_pooling2d_1 (MaxPooling2 (None, 5, 5, 32) 0
_________________________________________________________________
flatten (Flatten) (None, 800) 0
_________________________________________________________________
dropout (Dropout) (None, 800) 0
_________________________________________________________________
dense (Dense) (None, 10) 8010
=================================================================
Total params: 10,554
Trainable params: 10,554
Non-trainable params: 0
_________________________________________________________________
[[836 1 21 23 6 6 91 0 15 1]
[ 3 955 1 26 3 1 9 0 2 0]
[ 15 1 742 8 130 1 99 0 4 0]
[ 53 11 13 838 25 0 50 0 10 0]
[ 4 3 127 50 701 0 109 0 6 0]
[ 0 0 0 6 0 907 0 52 6 29]
[203 1 134 20 112 0 506 0 23 1]
[ 0 0 0 0 0 22 0 901 1 76]
[ 3 1 16 2 5 5 18 9 940 1]
[ 0 0 1 0 0 5 0 31 1 962]]
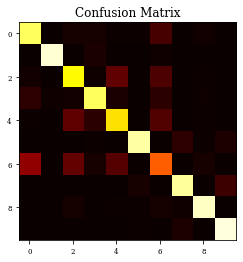
precision recall f1-score support
0 0.75 0.84 0.79 1000
1 0.98 0.95 0.97 1000
2 0.70 0.74 0.72 1000
3 0.86 0.84 0.85 1000
4 0.71 0.70 0.71 1000
5 0.96 0.91 0.93 1000
6 0.57 0.51 0.54 1000
7 0.91 0.90 0.90 1000
8 0.93 0.94 0.94 1000
9 0.90 0.96 0.93 1000
accuracy 0.83 10000
macro avg 0.83 0.83 0.83 10000
weighted avg 0.83 0.83 0.83 10000
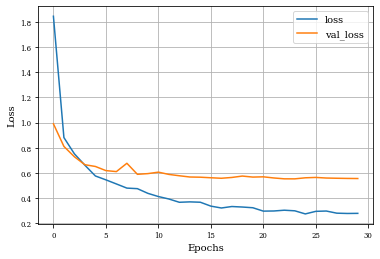
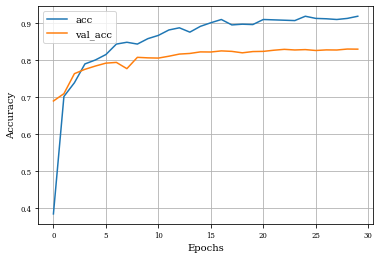
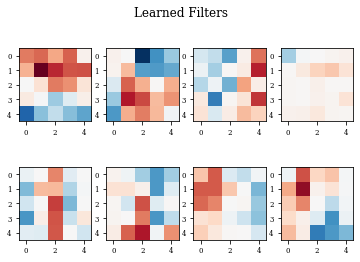
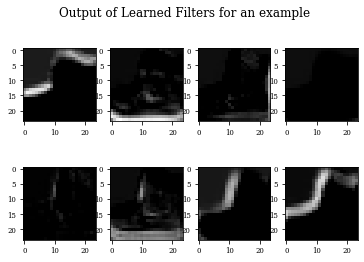
Example of Dilation Layer¶
histDil=see_results_layer(Dilation2D(nfilterstolearn, padding='valid',kernel_size=(filter_size, filter_size),kernel_regularization=L1L2Lattice(l1=regularizer_parameter,l2=regularizer_parameter)),lr=.01)
Model: "model_2"
_________________________________________________________________
Layer (type) Output Shape Param #
=================================================================
input_2 (InputLayer) [(None, 28, 28, 1)] 0
_________________________________________________________________
dilation2d (Dilation2D) (None, 24, 24, 8) 200
_________________________________________________________________
max_pooling2d_2 (MaxPooling2 (None, 12, 12, 8) 0
_________________________________________________________________
conv2d_2 (Conv2D) (None, 10, 10, 32) 2336
_________________________________________________________________
max_pooling2d_3 (MaxPooling2 (None, 5, 5, 32) 0
_________________________________________________________________
flatten_1 (Flatten) (None, 800) 0
_________________________________________________________________
dropout_1 (Dropout) (None, 800) 0
_________________________________________________________________
dense_1 (Dense) (None, 10) 8010
=================================================================
Total params: 10,546
Trainable params: 10,546
Non-trainable params: 0
_________________________________________________________________
[[802 12 30 35 10 3 99 1 8 0]
[ 11 915 12 35 12 0 12 0 3 0]
[ 11 2 670 8 195 0 106 0 8 0]
[ 54 59 13 780 41 0 46 0 7 0]
[ 5 7 133 50 689 1 108 0 7 0]
[ 0 0 0 2 0 824 0 89 9 76]
[214 13 216 40 162 0 331 0 24 0]
[ 0 0 0 0 0 43 0 885 3 69]
[ 0 1 54 8 4 23 8 14 888 0]
[ 0 0 0 1 0 12 0 40 4 943]]
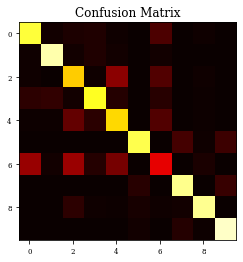
precision recall f1-score support
0 0.73 0.80 0.76 1000
1 0.91 0.92 0.91 1000
2 0.59 0.67 0.63 1000
3 0.81 0.78 0.80 1000
4 0.62 0.69 0.65 1000
5 0.91 0.82 0.86 1000
6 0.47 0.33 0.39 1000
7 0.86 0.89 0.87 1000
8 0.92 0.89 0.91 1000
9 0.87 0.94 0.90 1000
accuracy 0.77 10000
macro avg 0.77 0.77 0.77 10000
weighted avg 0.77 0.77 0.77 10000
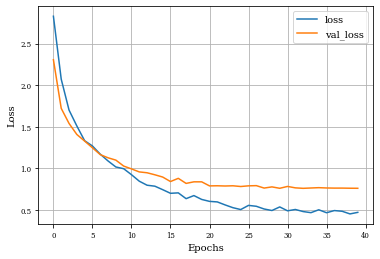
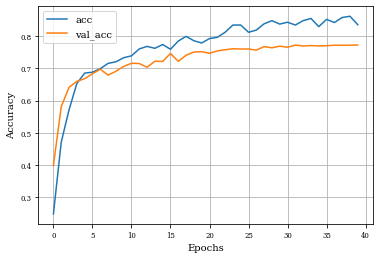
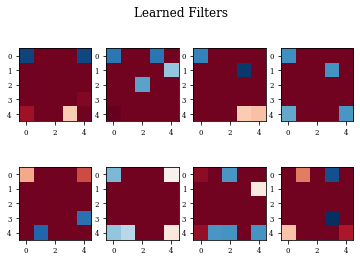
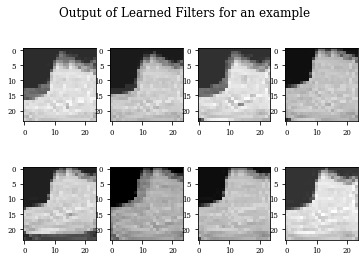
Example of Erosion Layer¶
histEro=see_results_layer(Erosion2D(nfilterstolearn, padding='valid',kernel_size=(filter_size, filter_size),kernel_regularization=L1L2Lattice(l1=regularizer_parameter,l2=regularizer_parameter)),lr=.01)
Model: "model_4"
_________________________________________________________________
Layer (type) Output Shape Param #
=================================================================
input_3 (InputLayer) [(None, 28, 28, 1)] 0
_________________________________________________________________
erosion2d (Erosion2D) (None, 24, 24, 8) 200
_________________________________________________________________
max_pooling2d_4 (MaxPooling2 (None, 12, 12, 8) 0
_________________________________________________________________
conv2d_3 (Conv2D) (None, 10, 10, 32) 2336
_________________________________________________________________
max_pooling2d_5 (MaxPooling2 (None, 5, 5, 32) 0
_________________________________________________________________
flatten_2 (Flatten) (None, 800) 0
_________________________________________________________________
dropout_2 (Dropout) (None, 800) 0
_________________________________________________________________
dense_2 (Dense) (None, 10) 8010
=================================================================
Total params: 10,546
Trainable params: 10,546
Non-trainable params: 0
_________________________________________________________________
[[785 1 39 34 5 1 123 0 12 0]
[ 7 953 0 29 6 0 5 0 0 0]
[ 10 3 658 10 187 0 130 0 2 0]
[ 50 27 28 808 32 0 48 0 7 0]
[ 0 3 108 51 705 3 121 0 9 0]
[ 0 0 0 4 0 879 1 62 8 46]
[187 5 129 38 126 0 495 0 20 0]
[ 0 0 0 0 0 33 0 881 1 85]
[ 2 2 16 3 5 19 32 9 912 0]
[ 0 1 0 2 0 3 2 45 0 947]]
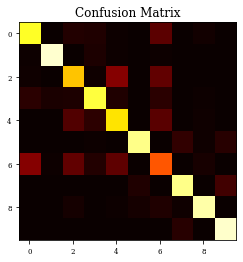
precision recall f1-score support
0 0.75 0.79 0.77 1000
1 0.96 0.95 0.96 1000
2 0.67 0.66 0.67 1000
3 0.83 0.81 0.82 1000
4 0.66 0.70 0.68 1000
5 0.94 0.88 0.91 1000
6 0.52 0.49 0.51 1000
7 0.88 0.88 0.88 1000
8 0.94 0.91 0.93 1000
9 0.88 0.95 0.91 1000
accuracy 0.80 10000
macro avg 0.80 0.80 0.80 10000
weighted avg 0.80 0.80 0.80 10000
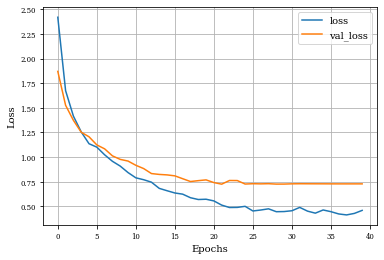
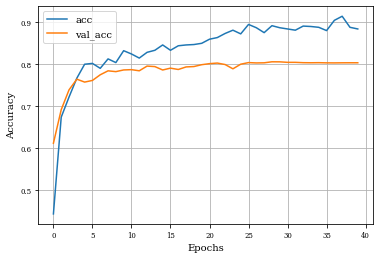
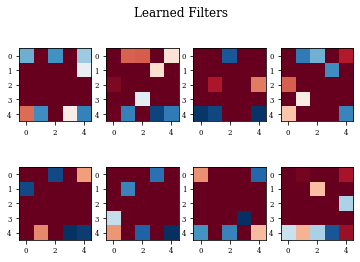
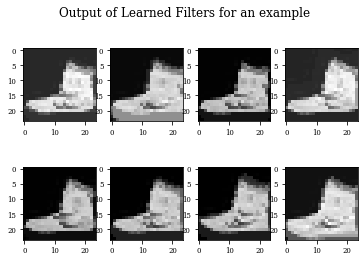
Example of Gradient Layer¶
histGrad=see_results_layer(Gradient2D(nfilterstolearn, padding='valid',kernel_size=(filter_size, filter_size),kernel_regularization=L1L2Lattice(l1=regularizer_parameter,l2=regularizer_parameter)),lr=.01)
Model: "model_6"
_________________________________________________________________
Layer (type) Output Shape Param #
=================================================================
input_4 (InputLayer) [(None, 28, 28, 1)] 0
_________________________________________________________________
gradient2d (Gradient2D) (None, 24, 24, 8) 200
_________________________________________________________________
max_pooling2d_6 (MaxPooling2 (None, 12, 12, 8) 0
_________________________________________________________________
conv2d_4 (Conv2D) (None, 10, 10, 32) 2336
_________________________________________________________________
max_pooling2d_7 (MaxPooling2 (None, 5, 5, 32) 0
_________________________________________________________________
flatten_3 (Flatten) (None, 800) 0
_________________________________________________________________
dropout_3 (Dropout) (None, 800) 0
_________________________________________________________________
dense_3 (Dense) (None, 10) 8010
=================================================================
Total params: 10,546
Trainable params: 10,546
Non-trainable params: 0
_________________________________________________________________
[[785 7 18 32 16 1 120 1 20 0]
[ 7 942 0 24 10 0 14 0 3 0]
[ 16 2 641 13 144 0 169 0 14 1]
[ 50 53 5 793 35 0 56 0 8 0]
[ 3 3 52 42 725 1 162 0 12 0]
[ 0 3 0 4 0 862 0 78 14 39]
[184 7 122 30 147 0 491 0 19 0]
[ 0 0 0 0 0 39 0 871 4 86]
[ 0 2 14 5 22 8 23 6 918 2]
[ 0 1 0 0 1 10 0 38 5 945]]
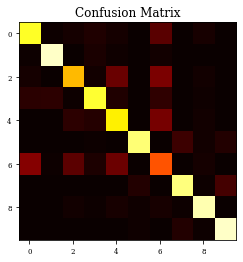
precision recall f1-score support
0 0.75 0.79 0.77 1000
1 0.92 0.94 0.93 1000
2 0.75 0.64 0.69 1000
3 0.84 0.79 0.82 1000
4 0.66 0.72 0.69 1000
5 0.94 0.86 0.90 1000
6 0.47 0.49 0.48 1000
7 0.88 0.87 0.87 1000
8 0.90 0.92 0.91 1000
9 0.88 0.94 0.91 1000
accuracy 0.80 10000
macro avg 0.80 0.80 0.80 10000
weighted avg 0.80 0.80 0.80 10000
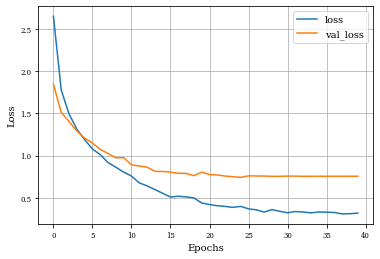
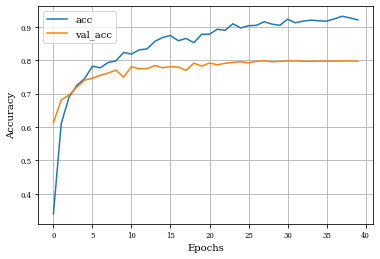
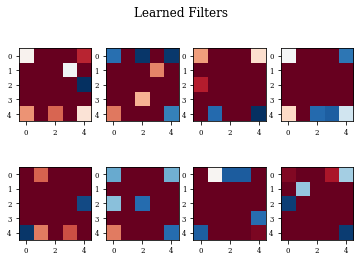
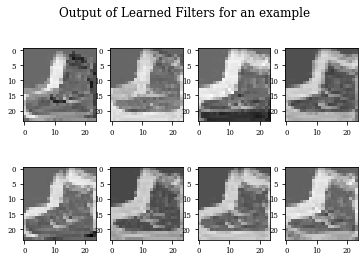
Example of Internal Gradient Layer¶
histInternalGrad=see_results_layer(InternalGradient2D(nfilterstolearn, padding='same',kernel_size=(filter_size, filter_size),kernel_regularization=L1L2Lattice(l1=regularizer_parameter,l2=regularizer_parameter)),lr=.01)
Model: "model_8"
_________________________________________________________________
Layer (type) Output Shape Param #
=================================================================
input_5 (InputLayer) [(None, 28, 28, 1)] 0
_________________________________________________________________
internal_gradient2d (Interna (None, 28, 28, 8) 200
_________________________________________________________________
max_pooling2d_8 (MaxPooling2 (None, 14, 14, 8) 0
_________________________________________________________________
conv2d_5 (Conv2D) (None, 12, 12, 32) 2336
_________________________________________________________________
max_pooling2d_9 (MaxPooling2 (None, 6, 6, 32) 0
_________________________________________________________________
flatten_4 (Flatten) (None, 1152) 0
_________________________________________________________________
dropout_4 (Dropout) (None, 1152) 0
_________________________________________________________________
dense_4 (Dense) (None, 10) 11530
=================================================================
Total params: 14,066
Trainable params: 14,066
Non-trainable params: 0
_________________________________________________________________
[[782 8 21 26 12 0 134 2 15 0]
[ 4 948 4 26 7 0 10 0 1 0]
[ 11 2 701 12 165 1 104 0 4 0]
[ 38 51 8 811 28 0 51 0 13 0]
[ 1 1 70 47 759 0 116 0 6 0]
[ 0 0 0 2 0 898 0 57 10 33]
[154 7 121 32 125 2 536 0 23 0]
[ 0 0 0 0 0 9 0 914 1 76]
[ 1 2 13 3 10 5 28 5 930 3]
[ 0 0 0 1 0 3 1 35 3 957]]
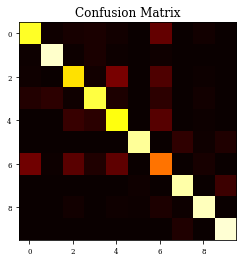
precision recall f1-score support
0 0.79 0.78 0.79 1000
1 0.93 0.95 0.94 1000
2 0.75 0.70 0.72 1000
3 0.84 0.81 0.83 1000
4 0.69 0.76 0.72 1000
5 0.98 0.90 0.94 1000
6 0.55 0.54 0.54 1000
7 0.90 0.91 0.91 1000
8 0.92 0.93 0.93 1000
9 0.90 0.96 0.93 1000
accuracy 0.82 10000
macro avg 0.82 0.82 0.82 10000
weighted avg 0.82 0.82 0.82 10000
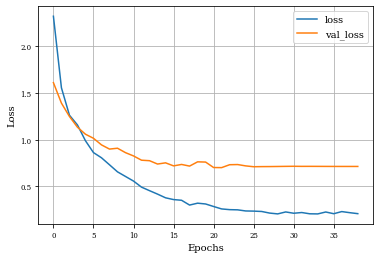
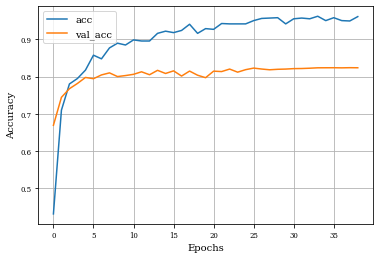
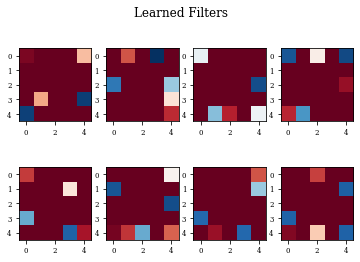
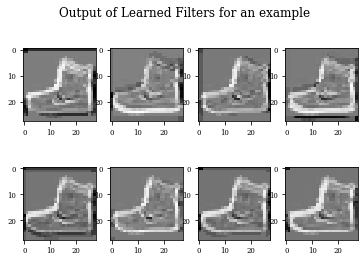
Example of Opening Layer¶
histOpening=see_results_layer(Opening2D(nfilterstolearn,padding='valid',kernel_size=(filter_size, filter_size),kernel_regularization=L1L2Lattice(l1=regularizer_parameter,l2=regularizer_parameter)),lr=.01)
Model: "model_10"
_________________________________________________________________
Layer (type) Output Shape Param #
=================================================================
input_6 (InputLayer) [(None, 28, 28, 1)] 0
_________________________________________________________________
opening2d (Opening2D) (None, 20, 20, 8) 200
_________________________________________________________________
max_pooling2d_10 (MaxPooling (None, 10, 10, 8) 0
_________________________________________________________________
conv2d_6 (Conv2D) (None, 8, 8, 32) 2336
_________________________________________________________________
max_pooling2d_11 (MaxPooling (None, 4, 4, 32) 0
_________________________________________________________________
flatten_5 (Flatten) (None, 512) 0
_________________________________________________________________
dropout_5 (Dropout) (None, 512) 0
_________________________________________________________________
dense_5 (Dense) (None, 10) 5130
=================================================================
Total params: 7,666
Trainable params: 7,666
Non-trainable params: 0
_________________________________________________________________
[[744 6 58 31 13 4 130 1 13 0]
[ 6 925 2 36 17 0 13 0 1 0]
[ 6 1 558 9 223 0 195 0 7 1]
[ 47 19 21 812 45 0 52 0 4 0]
[ 0 3 192 44 654 3 85 0 19 0]
[ 0 0 0 5 0 884 0 70 11 30]
[173 5 231 20 207 3 345 0 16 0]
[ 0 0 0 0 0 30 0 872 4 94]
[ 3 2 48 6 41 8 22 20 849 1]
[ 0 0 0 2 0 7 0 37 1 953]]
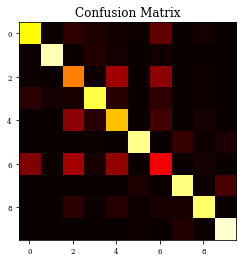
precision recall f1-score support
0 0.76 0.74 0.75 1000
1 0.96 0.93 0.94 1000
2 0.50 0.56 0.53 1000
3 0.84 0.81 0.83 1000
4 0.55 0.65 0.59 1000
5 0.94 0.88 0.91 1000
6 0.41 0.34 0.37 1000
7 0.87 0.87 0.87 1000
8 0.92 0.85 0.88 1000
9 0.88 0.95 0.92 1000
accuracy 0.76 10000
macro avg 0.76 0.76 0.76 10000
weighted avg 0.76 0.76 0.76 10000
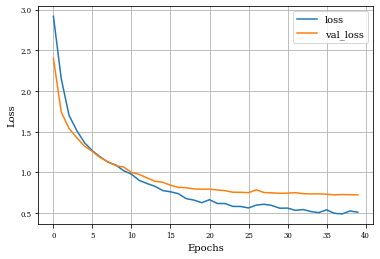
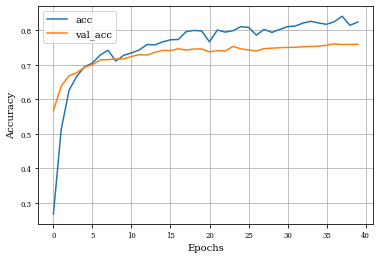
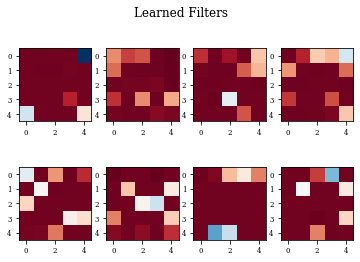
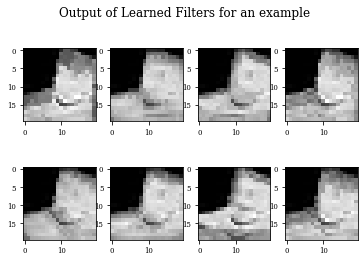
Example of Closing Layer¶
histClosing=see_results_layer(Closing2D(nfilterstolearn,padding='valid',kernel_size=(filter_size, filter_size),kernel_regularization=L1L2Lattice(l1=regularizer_parameter,l2=regularizer_parameter)),lr=.01)
Model: "model_12"
_________________________________________________________________
Layer (type) Output Shape Param #
=================================================================
input_7 (InputLayer) [(None, 28, 28, 1)] 0
_________________________________________________________________
closing2d (Closing2D) (None, 20, 20, 8) 200
_________________________________________________________________
max_pooling2d_12 (MaxPooling (None, 10, 10, 8) 0
_________________________________________________________________
conv2d_7 (Conv2D) (None, 8, 8, 32) 2336
_________________________________________________________________
max_pooling2d_13 (MaxPooling (None, 4, 4, 32) 0
_________________________________________________________________
flatten_6 (Flatten) (None, 512) 0
_________________________________________________________________
dropout_6 (Dropout) (None, 512) 0
_________________________________________________________________
dense_6 (Dense) (None, 10) 5130
=================================================================
Total params: 7,666
Trainable params: 7,666
Non-trainable params: 0
_________________________________________________________________
[[754 24 43 32 16 5 108 1 17 0]
[ 5 911 10 42 22 0 9 0 1 0]
[ 11 4 633 7 198 2 128 0 17 0]
[ 50 88 14 706 75 0 53 0 14 0]
[ 4 5 304 64 540 3 67 0 13 0]
[ 0 0 0 6 0 817 1 88 15 73]
[204 11 300 36 177 2 255 0 15 0]
[ 0 0 1 0 0 51 0 853 3 92]
[ 5 1 129 4 22 17 18 18 783 3]
[ 0 0 0 3 0 8 0 41 5 943]]
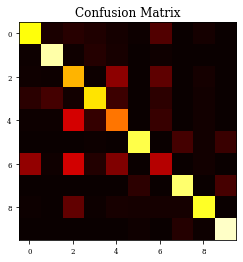
precision recall f1-score support
0 0.73 0.75 0.74 1000
1 0.87 0.91 0.89 1000
2 0.44 0.63 0.52 1000
3 0.78 0.71 0.74 1000
4 0.51 0.54 0.53 1000
5 0.90 0.82 0.86 1000
6 0.40 0.26 0.31 1000
7 0.85 0.85 0.85 1000
8 0.89 0.78 0.83 1000
9 0.85 0.94 0.89 1000
accuracy 0.72 10000
macro avg 0.72 0.72 0.72 10000
weighted avg 0.72 0.72 0.72 10000
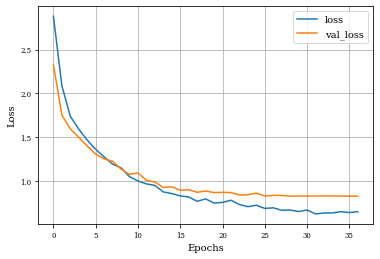
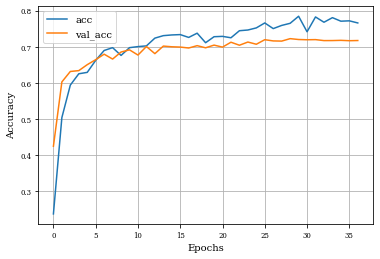
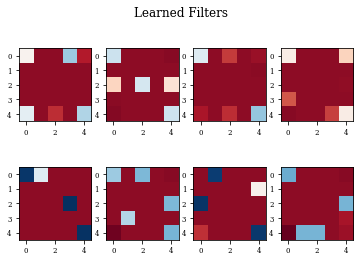
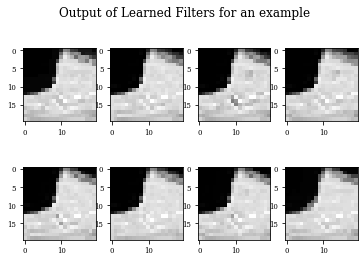
Example of Top Hat Opening Layer¶
histTopHatOpening=see_results_layer(TopHatOpening2D(nfilterstolearn,kernel_size=(filter_size, filter_size),kernel_regularization=L1L2Lattice(l1=regularizer_parameter,l2=regularizer_parameter)),lr=.01)
Model: "model_14"
_________________________________________________________________
Layer (type) Output Shape Param #
=================================================================
input_8 (InputLayer) [(None, 28, 28, 1)] 0
_________________________________________________________________
top_hat_opening2d (TopHatOpe (None, 28, 28, 8) 200
_________________________________________________________________
max_pooling2d_14 (MaxPooling (None, 14, 14, 8) 0
_________________________________________________________________
conv2d_8 (Conv2D) (None, 12, 12, 32) 2336
_________________________________________________________________
max_pooling2d_15 (MaxPooling (None, 6, 6, 32) 0
_________________________________________________________________
flatten_7 (Flatten) (None, 1152) 0
_________________________________________________________________
dropout_7 (Dropout) (None, 1152) 0
_________________________________________________________________
dense_7 (Dense) (None, 10) 11530
=================================================================
Total params: 14,066
Trainable params: 14,066
Non-trainable params: 0
_________________________________________________________________
[[780 10 26 38 5 1 112 4 23 1]
[ 3 934 3 40 7 0 10 0 3 0]
[ 14 1 673 13 146 1 140 0 12 0]
[ 51 25 6 824 23 0 50 0 21 0]
[ 7 0 85 58 671 1 166 0 12 0]
[ 0 0 0 1 0 893 1 59 9 37]
[167 3 137 47 100 0 518 0 28 0]
[ 0 0 0 0 0 30 0 884 2 84]
[ 6 5 5 6 11 4 12 4 945 2]
[ 0 0 0 0 0 6 3 34 3 954]]
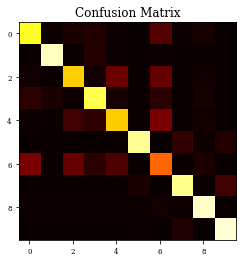
precision recall f1-score support
0 0.76 0.78 0.77 1000
1 0.96 0.93 0.94 1000
2 0.72 0.67 0.70 1000
3 0.80 0.82 0.81 1000
4 0.70 0.67 0.68 1000
5 0.95 0.89 0.92 1000
6 0.51 0.52 0.51 1000
7 0.90 0.88 0.89 1000
8 0.89 0.94 0.92 1000
9 0.88 0.95 0.92 1000
accuracy 0.81 10000
macro avg 0.81 0.81 0.81 10000
weighted avg 0.81 0.81 0.81 10000
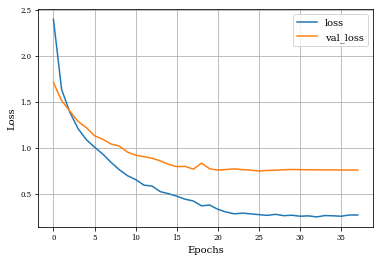
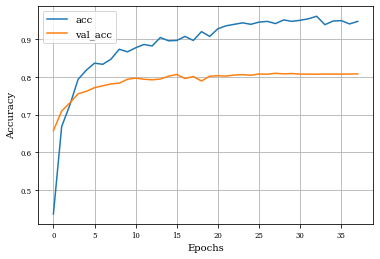
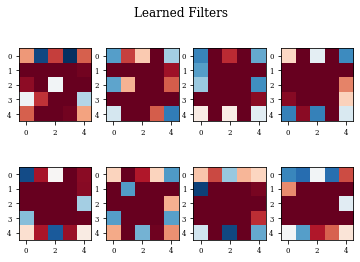
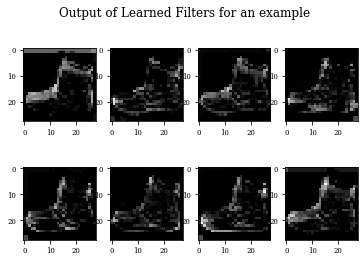
Example of Top Hat Closing Layer¶
histTopHatClosing=see_results_layer(TopHatClosing2D(nfilterstolearn,kernel_size=(filter_size, filter_size),kernel_regularization=L1L2Lattice(l1=regularizer_parameter,l2=regularizer_parameter)),lr=.01)
Model: "model_16"
_________________________________________________________________
Layer (type) Output Shape Param #
=================================================================
input_9 (InputLayer) [(None, 28, 28, 1)] 0
_________________________________________________________________
top_hat_closing2d (TopHatClo (None, 28, 28, 8) 200
_________________________________________________________________
max_pooling2d_16 (MaxPooling (None, 14, 14, 8) 0
_________________________________________________________________
conv2d_9 (Conv2D) (None, 12, 12, 32) 2336
_________________________________________________________________
max_pooling2d_17 (MaxPooling (None, 6, 6, 32) 0
_________________________________________________________________
flatten_8 (Flatten) (None, 1152) 0
_________________________________________________________________
dropout_8 (Dropout) (None, 1152) 0
_________________________________________________________________
dense_8 (Dense) (None, 10) 11530
=================================================================
Total params: 14,066
Trainable params: 14,066
Non-trainable params: 0
_________________________________________________________________
[[801 3 26 49 3 2 104 1 11 0]
[ 2 949 3 28 5 0 11 0 2 0]
[ 15 0 684 10 141 1 144 0 5 0]
[ 37 15 11 817 39 0 65 0 16 0]
[ 12 1 92 57 653 0 178 0 7 0]
[ 0 0 0 1 1 877 3 86 6 26]
[159 0 121 32 130 0 536 0 22 0]
[ 0 0 0 0 0 60 0 894 1 45]
[ 3 6 8 10 12 6 35 6 909 5]
[ 0 0 0 0 0 17 6 47 2 928]]
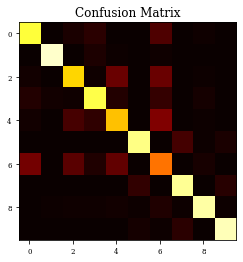
precision recall f1-score support
0 0.78 0.80 0.79 1000
1 0.97 0.95 0.96 1000
2 0.72 0.68 0.70 1000
3 0.81 0.82 0.82 1000
4 0.66 0.65 0.66 1000
5 0.91 0.88 0.89 1000
6 0.50 0.54 0.51 1000
7 0.86 0.89 0.88 1000
8 0.93 0.91 0.92 1000
9 0.92 0.93 0.93 1000
accuracy 0.80 10000
macro avg 0.81 0.80 0.81 10000
weighted avg 0.81 0.80 0.81 10000
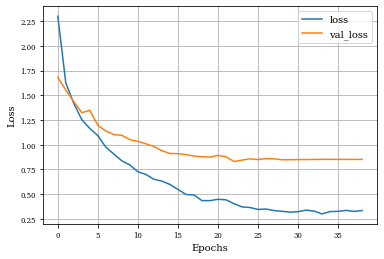
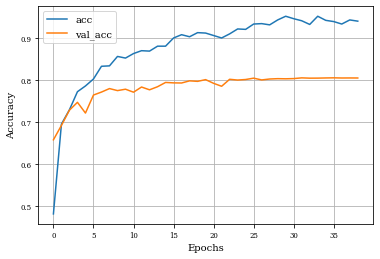
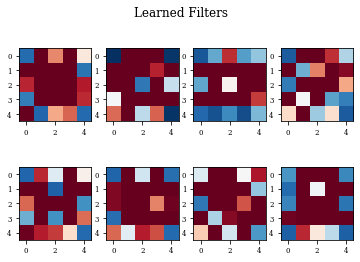
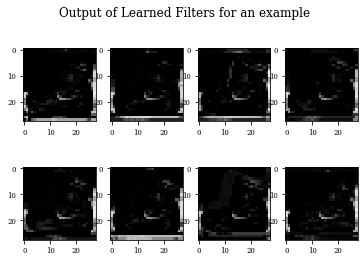
Example of Toggle Mapping Layer¶
histToggle=see_results_layer(ToggleMapping2D(nfilterstolearn,kernel_size=(filter_size, filter_size),kernel_regularization=L1L2Lattice(l1=regularizer_parameter,l2=regularizer_parameter)),lr=.01)
Model: "model_18"
_________________________________________________________________
Layer (type) Output Shape Param #
=================================================================
input_10 (InputLayer) [(None, 28, 28, 1)] 0
_________________________________________________________________
toggle_mapping2d (ToggleMapp (None, 28, 28, 8) 200
_________________________________________________________________
max_pooling2d_18 (MaxPooling (None, 14, 14, 8) 0
_________________________________________________________________
conv2d_10 (Conv2D) (None, 12, 12, 32) 2336
_________________________________________________________________
max_pooling2d_19 (MaxPooling (None, 6, 6, 32) 0
_________________________________________________________________
flatten_9 (Flatten) (None, 1152) 0
_________________________________________________________________
dropout_9 (Dropout) (None, 1152) 0
_________________________________________________________________
dense_9 (Dense) (None, 10) 11530
=================================================================
Total params: 14,066
Trainable params: 14,066
Non-trainable params: 0
_________________________________________________________________
[[771 10 21 30 14 3 132 2 17 0]
[ 4 945 4 30 7 0 7 0 3 0]
[ 8 2 686 13 189 0 95 0 7 0]
[ 50 60 8 777 33 0 64 0 8 0]
[ 1 8 91 53 710 0 132 0 5 0]
[ 1 0 0 0 0 874 0 65 10 50]
[171 5 138 33 127 0 504 0 22 0]
[ 0 0 0 0 0 20 0 904 1 75]
[ 3 4 14 5 5 3 35 6 925 0]
[ 0 0 1 1 0 6 2 39 2 949]]
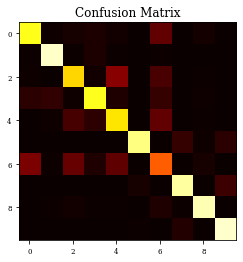
precision recall f1-score support
0 0.76 0.77 0.77 1000
1 0.91 0.94 0.93 1000
2 0.71 0.69 0.70 1000
3 0.82 0.78 0.80 1000
4 0.65 0.71 0.68 1000
5 0.96 0.87 0.92 1000
6 0.52 0.50 0.51 1000
7 0.89 0.90 0.90 1000
8 0.93 0.93 0.93 1000
9 0.88 0.95 0.92 1000
accuracy 0.80 10000
macro avg 0.81 0.80 0.80 10000
weighted avg 0.81 0.80 0.80 10000
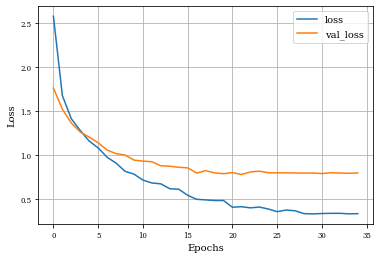
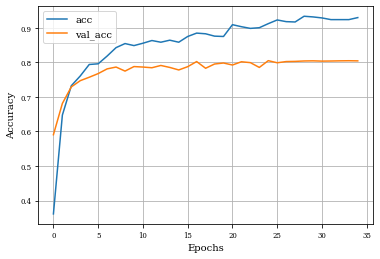
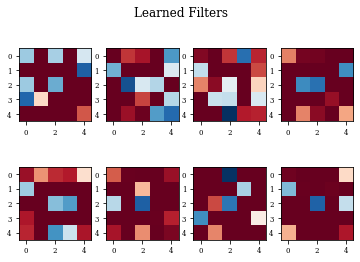
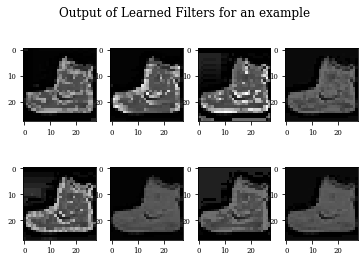
Summary of Results¶
Best Results for method in training accurary:
print('Linear Convolution: ',max(histConv.history['accuracy']))
print('Dilation: ',max(histDil.history['accuracy']))
print('Erosion: ',max(histEro.history['accuracy']))
print('Gradient: ',max(histGrad.history['accuracy']))
print('Internal Gradient: ',max(histInternalGrad.history['accuracy']))
print('Toggle: ',max(histToggle.history['accuracy']))
print('TopHatOpening: ',max(histTopHatOpening.history['accuracy']))
print('TopHatClosing: ',max(histTopHatClosing.history['accuracy']))
Linear Convolution: 0.9189453125
Dilation: 0.8623046875
Erosion: 0.9130859375
Gradient: 0.931640625
Internal Gradient: 0.9619140625
Toggle: 0.93359375
TopHatOpening: 0.9619140625
TopHatClosing: 0.9521484375
Best Results for method in validation accurary:
print('Linear Convolution: ',max(histConv.history['val_accuracy']))
print('Dilation: ',max(histDil.history['val_accuracy']))
print('Erosion: ',max(histEro.history['val_accuracy']))
print('Gradient: ',max(histGrad.history['val_accuracy']))
print('Internal Gradient: ',max(histInternalGrad.history['val_accuracy']))
print('Toggle: ',max(histToggle.history['val_accuracy']))
print('TopHatOpening: ',max(histTopHatOpening.history['val_accuracy']))
print('TopHatClosing: ',max(histTopHatClosing.history['val_accuracy']))
Linear Convolution: 0.830299973487854
Dilation: 0.7727000117301941
Erosin: 0.8046000003814697
Gradient: 0.7986000180244446
Internal Gradient: 0.8238999843597412
Toggle: 0.8050000071525574
TopHatOpening: 0.8100000023841858
TopHatClosing: 0.8054999709129333
Comparison of Validaciones accuracy
plt.figure(figsize=(20,20))
plt.plot(histConv.history['val_accuracy'],label='Linear Convolution')
plt.plot(histDil.history['val_accuracy'],label='Dilation')
plt.plot(histEro.history['val_accuracy'],label='Erosion')
plt.plot(histGrad.history['val_accuracy'],label='Gradient')
plt.plot(histInternalGrad.history['val_accuracy'],label='Internal Gradient')
plt.plot(histToggle.history['val_accuracy'],label='Toggle')
plt.plot(histTopHatOpening.history['val_accuracy'],label='TopHatOpening')
plt.plot(histTopHatClosing.history['val_accuracy'],label='TopHatClosing')
plt.xlabel('Epochs')
plt.ylabel('Validation Accuracy')
plt.grid()
plt.legend()
<matplotlib.legend.Legend at 0x1a4057b9b0>
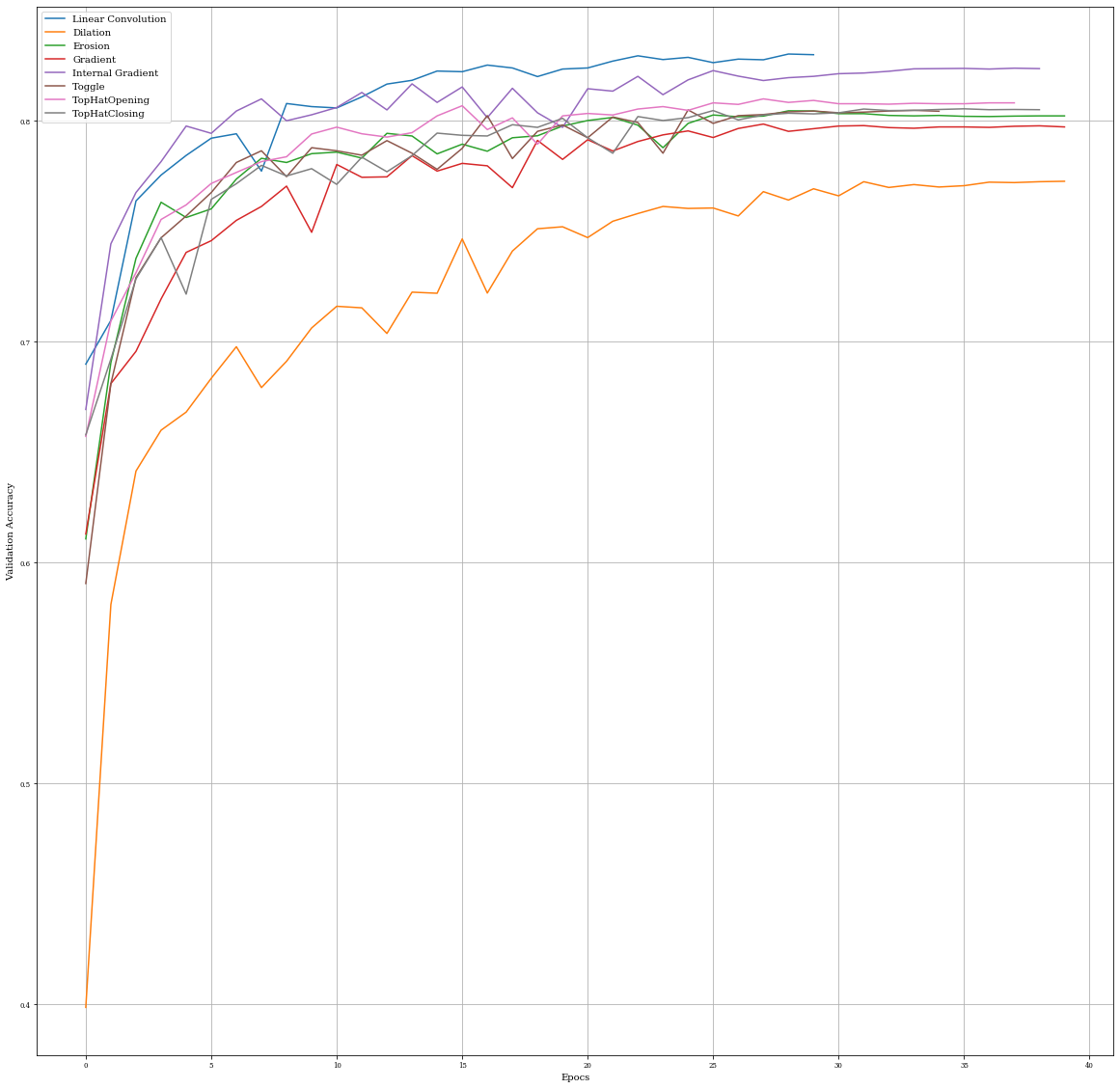